Baker: From Tracking to Classroom Instruction, Ed Tech Based on Biased Data Can Make Inequities Worse. What Designers and Engineers Can Do
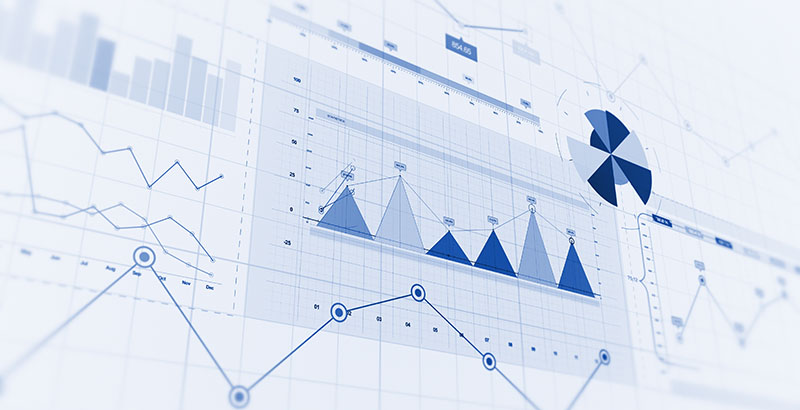
Get stories like these delivered straight to your inbox. Sign up for The 74 Newsletter
When COVID-19 forced schools to shut down in March 2020, many students in wealthier communities were able to adjust with high-speed home internet, plenty of resources and tutoring available in pandemic pods. It wasn’t perfect, but they got through it. Others, like students in rural Marion, Alabama, were stranded, unable to complete work without reliable internet.
Technology was a necessary part of education even before the pandemic. It has enormous promise and the potential to reduce racial and income-based inequities by making the best resources more widely available. But in practice, technology — as the digital divide in internet access makes clear — can also exacerbate existing disparities. And new technologies, especially those based on algorithms and artificial intelligence, give rise to serious questions of bias.
Ed tech developers, and the discipline of learning engineering as a whole, must pay close attention to these dangers as they leverage artificial intelligence and other new technology to produce better learning tools. This means making sure efforts to eliminate bias and provide equitable access are built into design principles and development.
On its face, learning technology seems like it should be neutral. Ed tech, the thinking goes, doesn’t know the background of the student using it, so how can it be biased? The answer lies in the assumptions that go into the design and implementation of these technologies. If interventions aren’t specifically designed with the varying experiences of disadvantaged students in mind, the default is likely to be systems that don’t work as well for those learners.
AI-based educational technologies can end up entrenching rather than disrupting inequities. If, for example, an AI technology designed to track students into different curricular programs is developed using data based on biased decisions, where underrepresented students are given less opportunity to excel, it may push low-achieving students onto tracks that ensure they aren’t challenged and remain deprived of enrichment opportunities.
Overreliance on learning technology can be a threat to equity, as well. Schools with budgetary difficulties may resort to using ed tech as a replacement for teacher-led instruction, instead of the supplement or complement it should be. One Mississippi school district, for example, has relied exclusively on online learning platforms for subjects like geometry. Solutions that simply put iPads and high-end technology in the hands of struggling students, without dedicated instruction and a detailed sense of what these students need, are likely to fail.
Equity-based learning engineering should take exactly the opposite approach. One-size-fits-all solutions tend to actually fit only one set of students, typically the most advantaged. Technology is generally designed with them in mind, not those students who exist on the margins, have had a less structured early education with fewer advantages and often have experienced some form of trauma.
It’s essential for learning engineers and ed tech designers to create and research interventions that are sensitive to the differences among learners and the needs of a range of disadvantaged student populations. This means getting away from a top-down way of designing that simply identifies abstract learning principles and integrates them into the newest technology. The impetus for ed tech design must come from the needs on the ground, in the particular learning environment in which the tools will be used.
By moving away from “the fallacy of the ‘average’” student, data-driven educational technologies can locate struggling kids quickly and personalize interventions. For example, new writing feedback technology can give students personalized responses and help direct teacher attention where it’s needed, so students recover from setbacks quickly. Since underserved students tend to face more barriers and are often forced to adjust to new environments, these tools can become important contributors to a more equitable education system.
Similarly, algorithms based on convenience samples — information taken from easy-to-reach student populations — can be less effective for specific groups of learners. And concerns around privacy can often encourage learning platform developers not to collect the types of demographic data necessary to be sure that technology is not biased.
Ed tech engineers must develop best practices to ensure that research into learning behavior and success draws from an appropriately diverse range of student populations, including with respect to race, ethnicity, language, gender, geography, neurodiversity and disabilities. Learning engineers also must do more to develop tests to ensure tools and algorithms are actually having their intended effects on given student populations.
Technology has made possible a whole new wave of learning tools, educational research and pedagogies. But it is not at all a given that these tools will be used to ameliorate longstanding, and in some cases worsening, problems and inequities. Only with the right principles and practices in place will education technology serve the students who need it most.
Ryan Baker is a professor of education at the University of Pennsylvania, where he directs the Penn Center for Learning Analytics. He is editor of the journal Computer-Based Learning in Context and associate editor of the Journal of Educational Data Mining.
Get stories like these delivered straight to your inbox. Sign up for The 74 Newsletter