Personalized Education Is Not a Panacea. Neither Is Artificial Intelligence
Kucirkova: Schools may want to jump on the AI bandwagon, but they must be careful that technologies don't perpetuate personalized learning's downsides
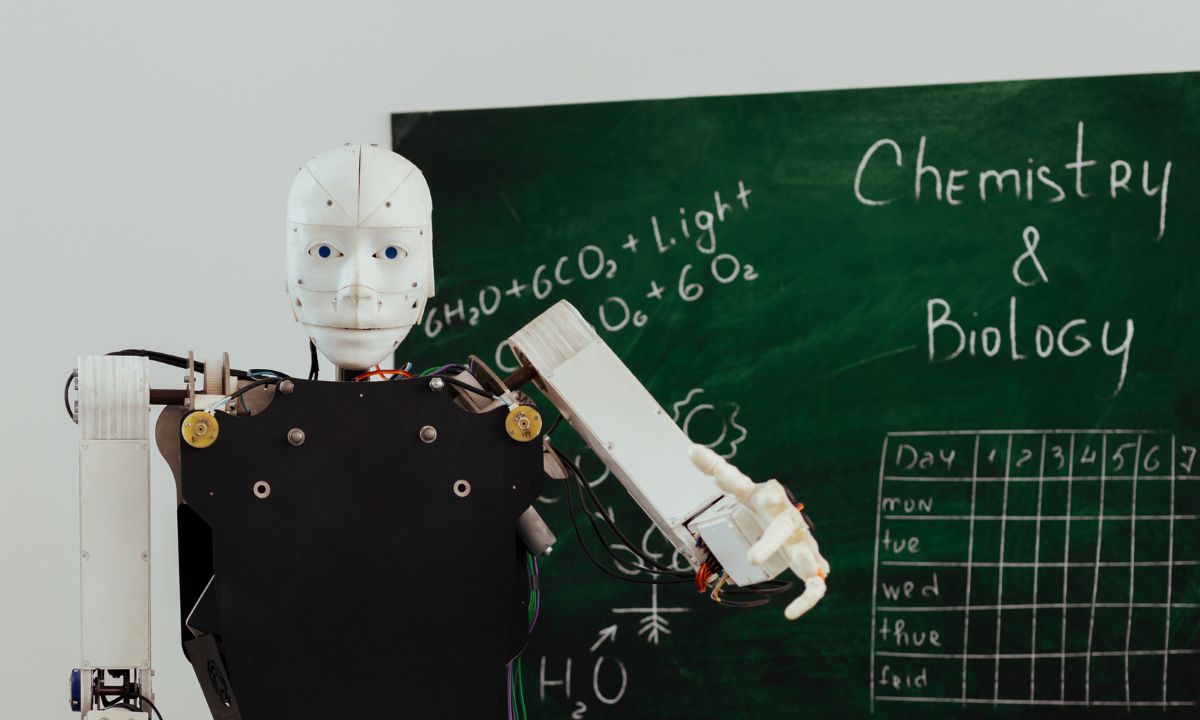
Get stories like this delivered straight to your inbox. Sign up for The 74 Newsletter
Many believe artificial intelligence can transform education, among them John Bailey, who argues that AI tutors and teaching assistants can accelerate personalized learning. However, personalized learning is not a panacea.
As a professor of early childhood, I have examined the impact of personalization on learning and social relationships. A personalized education can be beneficial: Educators who move away from rigid, standardized approaches support the diverse strengths and potentials of every student. But it also has downsides, and AI can accelerate these. Attention must be paid to ensuring AI does not deepen existing biases or take away the possibility for students to make choices and decisions about what they learn.
There are three mistaken assumptions that must be avoided when using AI for learning.
First is the assumption that increasing personalization will always translate into improved learning. Yes, targeted feedback in the form of corrections and questions can support students’ progress from one level to the next, and personalized applications can target the difficulty of a task. In this way, personalization makes learning easier. The problem: People often learn best when faced with a difficult challenge. “No pain, no gain” is a real thing.
Babies learn about the world by making discoveries, analyzing patterns and forming predictions. Like scientists, they learn most when their expectations get violated. Effective education is driven by surprise and challenges.
Personalization can engage students in learning, but engagement alone is not enough. For learning to stick, students need to be pushed to work harder and think deeper. Put simply, this means combining personalization with diversification. Good teachers focus on the student and the class simultaneously: Both the individual and the collective matter. In practice, this means some content is purposefully tailored to the child’s progress and some is not. In contrast, personalized AI tutors support only individual learning. Instead, they should apply personalized learning in small doses and in strategic moments, rather than use personalization as an umbrella approach.
The second assumption is that personalized education provides students with more choice than standardized approaches do. But people often forget that it matters who is doing the choosing. There is a difference between the learner making the choice and automatic personalization. Amazon’s personalized shopping recommendations are an example of automatic personalization, with minimal user agency.
Agency may not matter for commercial goals, but it does for effective learning. Educational technologies should give students a choice in how and what they learn, though not all the time. Effective learning happens when there is a balance between allowing students to freely choose and automatically assigning optimal content to them.
AI applications also need to be transparent; this is especially important when students’ agency is involved. For students to make appropriate choices for themselves and teachers to make proper choices for their students, they need to know how AI algorithms make decisions and which data they use. With access to the data the AI system has at its disposal and the data that they feed into the system, students and teachers can better decide whether to accept a personalized recommendation generated by AI.
The third, somewhat paradoxical, assumption is that personalized AI education will solve educational inequity. Providing students with individualized feedback is certainly hard to achieve in large classes, and AI can help schools compensate for teacher shortages. However, personalized AI tutors are being introduced into an inherently unequal education system that often discriminates against certain ethnicities, genders and disabilities. Just as early applications of personalized medicine were criticized for disadvantaging underprivileged people and ethnic minorities, so does personalized education.
The gap between white and minority students will not automatically narrow through use of personalized AI tutors. Standardized assessments that guide teachers in monitoring student outcomes and prescribed, structured teaching remain essential for high-needs students. Schools may want to jump on the AI bandwagon, but they need to be careful that technologies do not propagate the downsides of personalized education.
Get stories like these delivered straight to your inbox. Sign up for The 74 Newsletter