How AI Can Help Create Assessments that Enhance Opportunities for all Students
Hamilton & Middlebrook: Educators can use artificial intelligence to make tests more learner-centered and personalized, with a whole-child emphasis.
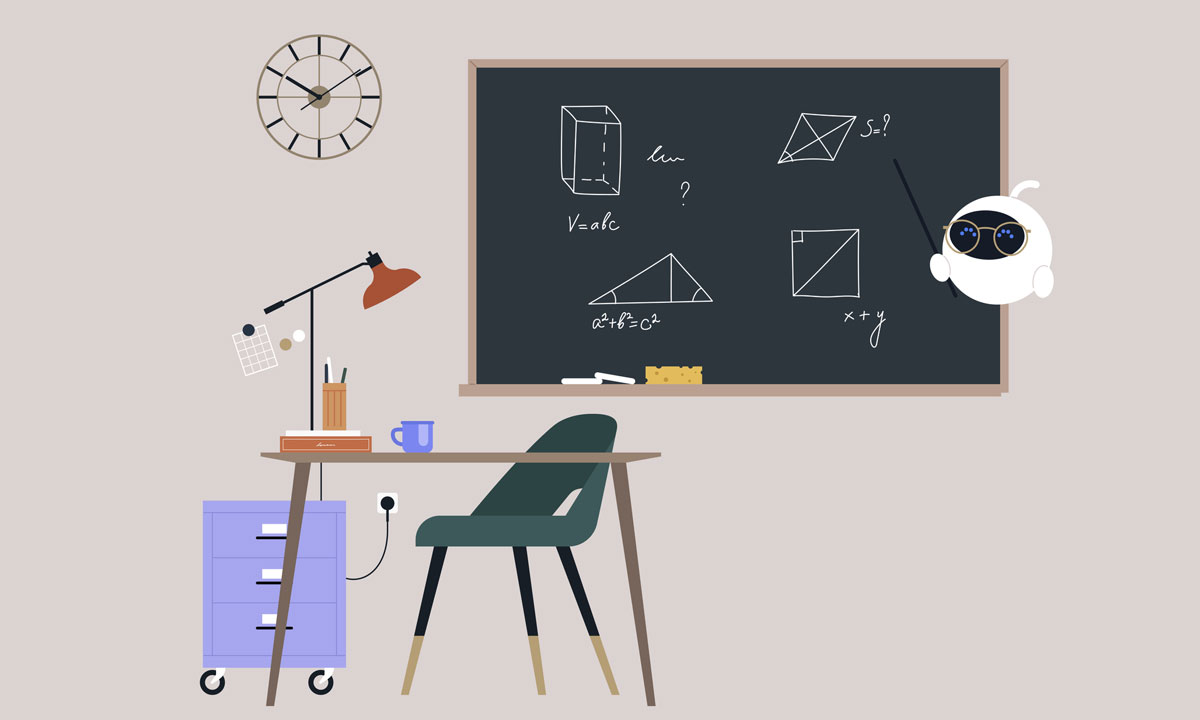
Get stories like this delivered straight to your inbox. Sign up for The 74 Newsletter
Like so many aspects of K-12 education, including classroom instruction, assessments of student learning are experiencing some titanic shifts. Two of the biggest factors driving these changes are the advancement of artificial intelligence tools and a growing commitment to the development of exams that improve opportunities for all students.
Developers are increasingly leveraging AI in assessment design, development, scoring and reporting. The implications include potential improvements that give real-time feedback and increase instructional efficiency. But there are also potential threats, such as algorithmic bias, so-called hallucinatory responses and increased surveillance that could weaken privacy protections.
Of course, advances in AI are not the only factor influencing the future of assessments. Inequities in educational opportunity are widespread, and professionals increasingly acknowledge that the use of tests for purposes ranging from college admissions to school accountability has largely failed to mitigate them. In response to this failure, exam developers, policymakers, community leaders and educators have argued for tools, practices and policies designed with the goal of enhancing opportunities for all learners.
These two trends offer a framework for a new approach that capitalizes on the promise of AI in ways that could benefit all students. We propose that such a paradigm should incorporate five key features.
- An emphasis on a whole-child, integrated view of learning and assessment. The Science of Learning and Development, based on decades of research, points to the integrated nature of academic, social and emotional development. AI-enhanced tools could emphasize this in several ways, such as by supporting the measurement of collaborative problem-solving skills or building digital measures of student engagement.
- A broader perspective on personalization. The phrases “personalized learning” and “personalized assessment” often emphasize adjusting instruction or exam content in response to student achievement and interests. As developers enact AI-driven personalization of assessment, they should explore opportunities to tailor assessment tasks not only to students’ prior achievement and interests, but also to their linguistic, social and cultural backgrounds.
- Reconsideration of how schools define and prioritize outcomes. AI is capable of performing jobs that have traditionally been carried out by humans. What, then, does it mean to demonstrate proficiency in writing when nearly everyone has a chatbot in their pocket? What kinds of media literacy and critical thinking skills do people need to navigate this changing landscape? To succeed in the modern workforce and flourish as adults, students will need to build proficiency across AI-related skills, and schools will need to figure out how to teach and assess them.
- A revised concept of test security. Along similar lines, concerns about how tools like ChatGPT might enable students to cheat are widespread. A learner-centered approach to assessment should acknowledge ways in which technology is advancing and what it means to be proficient in affected areas, such as research and writing. This approach should also consider how to incorporate AI tools into assessment tasks, rather than treating them as threats to the accuracy of resulting test scores.
- Prioritization of human relationships. Research documents the value of supportive relationships and a sense of belonging in schools, and thoughtful commentaries on the role of AI in education have emphasized the need to maintain human connections. This advice applies equally to assessment: Despite the potential improvements to quality and efficiency stemming from automation of test development, scoring and reporting, human involvement in the process can provide valuable opportunities for connection and collaborative learning. Additionally, digital measures of engagement, collaboration and other aspects of student development provide only partial information and should be supplemented with educator and peer input.
The integration of AI into educational assessments that are learner-centered will bring potential benefits and pitfalls. For instance, new tests that incorporate a whole-child perspective could generate useful evidence to inform instruction, but they could also result in inappropriate inferences about students’ capabilities or raise concerns among parents or others with objections to the teaching of social and emotional learning. Similarly, research on personalized learning makes it clear that state and local policies, along with supports for teachers such as professional development, will need to be aligned with the goal of personalization.
Achieving the vision of a learner-centered assessment system that leverages the best of modern technology will require a collaborative approach that involves research and development teams, policymakers, educators and, perhaps most importantly, the young people who have the greatest stake in how this work evolves. All these groups must keep their collective emphasis on the ultimate goal — measuring what truly contributes to the holistic development of each student while ensuring that the human perspective and unique experiences of educators and learners remain at the center.
Get stories like these delivered straight to your inbox. Sign up for The 74 Newsletter