Heffernan: Some Say the U.S. Has Lost the AI Race to China, but There’s Still Time to Catch Up — If We Start Teaching Data Science & Literacy in Schools
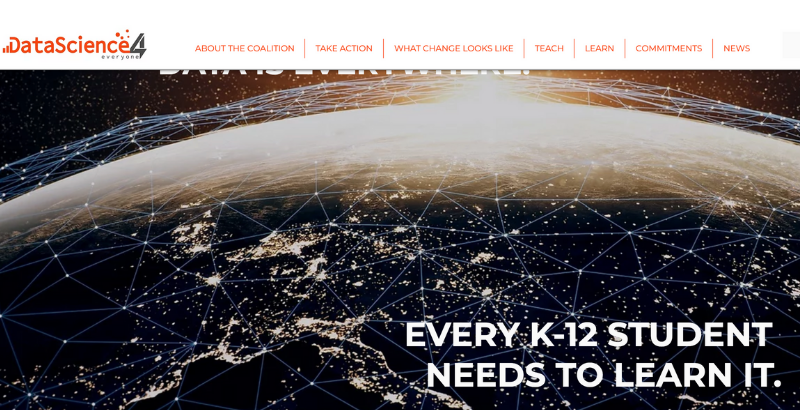
Get stories like these delivered straight to your inbox. Sign up for The 74 Newsletter
China has posted meteoric growth in technology over the last decade and, in recent years, made tremendous advances in natural language processing, machine learning and big data. Some observers say the U.S. has already lost the artificial intelligence race and point to some potentially dramatic national security implications.
But the AI race is still in its early stages, and America has time to reverse the trend, especially if it focuses on the data science education needed to produce technological breakthroughs, educate the workforce and give people the tools to understand the world around them.
The stakes are high. AI technology is quickly becoming the backbone of economic advancement globally. From weapons development to food production to health care, America’s ability to advance AI technology will determine its future as a global superpower.
Data literacy is not just needed among cutting-edge experts and specialists. The jobs of the future are becoming increasingly technical at every level. Advances in technology have drastically changed how we engage with numbers and mathematics on a daily basis — thinking through issues like COVID-19, climate change or increasing income inequality requires a basic understanding of how to analyze and interpret data. Countries with populations unable to understand and work with data are a severe disadvantage globally.
Expert recommendations for closing the AI gap between the U.S. and China’s focus on high-level skills. According to a report from the National Security Commission on AI, “The human talent deficit is the government’s most conspicuous AI deficit and the single greatest inhibitor to buying, building and fielding AI-enabled technologies for national security purposes.”
Developing this talent to compete must start with basic skills in data analysis, statistics and modeling at the K-12 level. Students need math fluency grounded in data science and computational thinking in order to develop and understand AI and related technologies and trends.
But data literacy isn’t being taught, and American math education in general is in a sorry state. Those of us who work in the education sector have a front-row seat to the many ways children, especially those from low-income and disadvantaged groups, are unprepared for the math future. Internationally, the U.S. lags behind other countries on the Program for International Student Assessment, ranking 36th out of 79 industrialized nations. China is ranked first.
Meanwhile, significant math learning gaps persist between white and high-income students, on the one hand, and low-income, Black and Hispanic students on the other. The 2019 National Assessment of Educational Progress revealed a 31-point math score disparity between white and Black 12th graders — unchanged since the 2005 NAEP.
Layer on the devastating impacts of the pandemic, and a perfect storm is brewing. Right now, on average, students are five months behind in mathematics, and opportunity and achievement gaps have only widened.
Math reform has had a long and somewhat checkered history in America, with its roots in Cold-War-era needs. The 1958 National Defense Education Act, signed by President Dwight Eisenhower in response to the Soviet launch of the Sputnik satellite, renewed and improved American math and science education. But it also left us with a secondary math curriculum that is ill-suited to modern needs. We need a similar effort to reorient and reinvigorate math instruction in response to contemporary challenges.
Common Core standards have gone part of the way, even if implementation has been a mixed success. A focus on data science literacy in math education can help build on this progress in providing engaging instruction that improves students’ skills in mathematical reasoning, while advancing American competitiveness and ingenuity.
Proficiency in data analysis requires both technical know-how, as well as higher-order conceptual and contextual thinking about what numbers mean. This presents an extraordinary opportunity for teachers. First of all, real-world connections — the absence of which are often discouraging to math students — are built in to data science. A teacher doesn’t need to explain the practical implications of a regression analysis of political polling data or upsets during March Madness. It’s immediately clear how the math helps us understand and analyze things we care about.
Secondly, because of these real-world connections, data science education can have a long-term effect on students’ intellectual development and future prospects, no matter what field they eventually go into. Students equipped with data science skills will think at a deeper level about how to apply mathematics to the problems that interest them.
From a global perspective, this means workers with needed skills in every field; companies with the talent to develop and maintain game-changing new technologies; and government with the resources needed to maintain America’s national security and international standing.
Although data science isn’t widely taught at the moment, there are some signs of change. San Diego and New York City schools are incorporating data science into their math curricula. At the state level, Virginia, Oregon and California are all including options for data science coursework as they revise their mathematics frameworks. Moreover, over 800 districts, states and organizations like my own have joined the Data Science for Everyone coalition to collectively advance these opportunities. The coalition’s annual Commitments Campaign, launching again in March, brings even more actors to the table to spotlight the change being made.
The U.S. needs a larger national movement toward increased data science and data literacy. Resources such as CourseKata and Bootstrap already integrate data science into math and all other subject areas. But other content and curriculum developers can do much more to infuse data science concepts into their core instructional materials to reach more students faster. Making data science a priority for school districts and states could create incentives for developers to fill the demand. In addition to in-class materials, digital learning tools and platforms focused on data science should be developed to help ensure that these resources are widely available. Finally, new professional learning programs are needed to support educators in teaching these skills.
We are in the midst of a tremendous technology-aided shift in how we do business, form public policy and compete internationally. This presents challenges and threats — and right now, American students are being left behind and outpaced by competitors like China. But it also presents opportunities, including the opportunity to change the way we teach math in order to help our children achieve prosperous futures.
Neil Heffernan is a professor of computer science at Worcester Polytechnic Institute and co-founder of ASSISTments.
Get stories like these delivered straight to your inbox. Sign up for The 74 Newsletter