AI Shows Racial Bias When Grading Essays — and Can’t Tell Good Writing From Bad
Smith: Study finds ChatGPT replicates human prejudices and fails to recognize exceptional work — reinforcing the inequalities it's intended to fix.
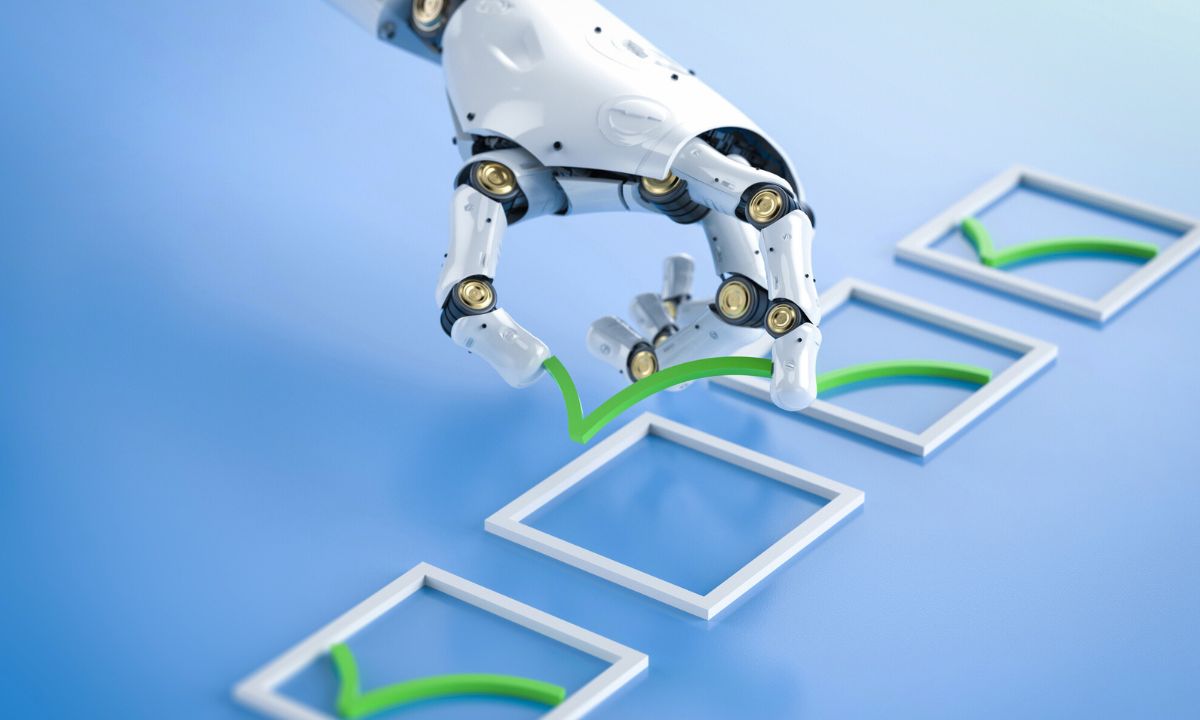
Get stories like this delivered straight to your inbox. Sign up for The 74 Newsletter
Every day, artificial intelligence reaches deeper into the nation’s classrooms, helping teachers personalize learning, tutor students and develop lesson plans. But the jury is still out on how well it does some of those jobs, notably grading student writing. A new study from The Learning Agency found that while ChatGPT can mimic human scoring when it comes to essays, it struggles to distinguish good writing from bad. And that has serious implications for students.
To better understand those implications, we evaluated ChatGPT’s essay scoring ability using the Automated Student Assessment Prize (ASAP) 2.0 benchmark. This includes approximately 24,000 argumentative essays written by U.S. middle and high school students. What makes ASAP 2.0 particularly useful for this type of research is that each essay was scored by humans, and it includes demographic data, such as race, English learner status, gender and the economic status of each student author. That means researchers can look at how AI performs not just in comparison to human scorers, but across different student groups.
So what did we find? Chat GPT did assign different average scores to different demographic groups, but most of those differences were so small, they probably wouldn’t matter much. However, there was one exception: Black students received lower scores than Asian students, and that gap was large enough to warrant some attention.
But here’s the thing: This same disparity appeared in human-assigned scores. In other words, ChatGPT didn’t introduce new bias, but rather replicated the bias that already existed in the human scoring data. While that might suggest the model accurately reflects current standards, it also highlights a serious risk. When training data reflects existing demographic disparities, those inequalities can be baked into the model itself. The result is then predictable: The same students who’ve historically been overlooked stay overlooked.
And that matters a lot. If AI models reinforce existing scoring disparities, students could see lower grades not because of poor writing, but because of how performance has been historically judged. Over time, this could impact academic confidence, access to advanced coursework or even college admissions, amplifying educational inequities rather than closing them.
Furthermore, our study also found that ChatGPT struggles to tell the difference between great and poor writing. Unlike human graders, who gave out more As and Fs, ChatGPT handed out a lot of Cs. That means strong writers may not get the recognition they deserve, while weaker writing could go unchecked. For students of marginalized backgrounds who often have to work harder to be noticed, that’s potentially a serious loss.
To be clear, human grading isn’t perfect. Teachers can harbor unconscious biases or apply inconsistent standards when scoring essays. But if AI both replicates those biases and fails to recognize exceptional work, it doesn’t fix the problem. It reinforces the same inequalities that so many advocates and educators are trying to fix.
That’s why schools and educators must carefully consider when and how to utilize AI for scoring. Rather than replacing grading, they could provide feedback on grammar or paragraph structure while leaving the final assessment to the teacher. Meanwhile, ed tech developers have a responsibility to evaluate their tools critically. It’s not enough to measure accuracy; developers need to ask: Who is it accurate for, and under what circumstances? Who benefits and who gets left behind?
Benchmark datasets like ASAP 2.0, which include demographic details and human scores, are essential for anyone trying to evaluate fairness in an AI system. But there is a need for more. Developers need access to more high-quality datasets, researchers need the funding to create them and the industry needs clear guidelines that prioritize equity from the start, not as an afterthought.
AI is beginning to reshape how students are taught and judged. But if that future is going to be fair, developers must build AI tools that account for bias, and educators must use them with clear boundaries in place. These tools should help all students shine, not flatten their potential to fit the average. The promise of educational AI isn’t just about efficiency. It’s about equity. And nobody can afford to get that part wrong.
Get stories like these delivered straight to your inbox. Sign up for The 74 Newsletter